April 22, 2025 • Nelson Cicchitto
The Role of AI-Driven Anomaly Detection in IAM: Revolutionizing Security Through Intelligent Monitoring
Discover how AI-driven anomaly detection is transforming access management, enabling organizations to detect threats in real-time
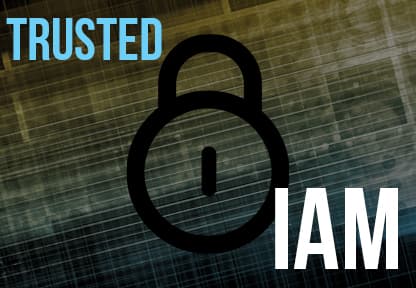
Traditional rule-based security approaches are increasingly insufficient to protect enterprise assets. As organizations migrate to cloud environments and embrace hybrid workforces, identity has become the new security perimeter. According to Gartner, by 2025, 75% of security failures will result from inadequate management of identities, access, and privileges – an increase from 50% in 2023.
This shifting paradigm demands more sophisticated identity and access management (IAM) solutions that can proactively identify threats before they materialize. AI-driven anomaly detection represents a transformative advancement in IAM technology, enabling security teams to move beyond reactive approaches to identity security.
Understanding AI-Driven Anomaly Detection in IAM
AI-driven anomaly detection in identity and access management leverages advanced algorithms and machine learning to establish baseline behavior patterns for users and systems. These intelligent systems continuously analyze access patterns, login behaviors, resource usage, and other identity-related activities to identify deviations that may indicate security threats.
Unlike traditional rule-based approaches that rely on predefined parameters, AI-powered anomaly detection can:
- Learn normal behavior patterns for individual users and user groups
- Adapt to changing working patterns and organizational shifts
- Detect subtle deviations that wouldn’t trigger conventional security alerts
- Reduce false positives by understanding contextual factors
- Continuously improve threat detection through machine learning
The Critical Need for Intelligent Anomaly Detection
The expanding digital attack surface and sophistication of modern threats have made AI-driven anomaly detection an essential component of modern identity management architecture. Consider these compelling statistics:
- Organizations experience an average of 2,200 identity-related security incidents annually, costing an average of $9.8 million, according to the Ponemon Institute’s 2023 Cost of Insider Threats Global Report.
- A recent study by Verizon found that 61% of data breaches involve credential abuse.
- According to IBM’s Cost of a Data Breach Report 2023, organizations with advanced security AI and automation experience breach costs of $3.05 million less than those without such capabilities.
Traditional IAM systems that rely solely on static rules and periodic reviews are increasingly vulnerable to sophisticated attacks that exploit legitimate credentials. AI-driven anomaly detection addresses these limitations by providing continuous, adaptive monitoring that can identify even the most subtle indicators of compromise.
Key Capabilities of AI-Driven Anomaly Detection
1. User Behavior Analytics (UBA)
UBA establishes behavioral baselines for each user and can detect anomalies such as:
- Unusual login times or locations
- Abnormal resource access patterns
- Suspicious privilege escalation
- Unusual data access or transfer volumes
- Deviations from typical workflow patterns
For example, if a sales executive who typically accesses CRM data during business hours suddenly begins downloading sensitive financial records at 2 AM from an overseas location, an AI-driven system would immediately flag this behavior for investigation.
2. Entity Behavior Analytics (EBA)
Beyond individual users, AI systems can monitor entity behavior, including:
- Application access patterns
- System-to-system communications
- API usage and data flows
- Service account activities
- Network traffic patterns
This broader perspective enables detection of sophisticated attacks that may involve multiple systems or accounts, such as lateral movement techniques used in advanced persistent threats.
3. Contextual Authentication
AI-driven anomaly detection enhances authentication by considering contextual factors:
- Geographic location and travel patterns
- Device characteristics and health
- Network characteristics
- Time patterns and work schedules
- Previous access history
By analyzing these factors in real-time, multifactor authentication systems can dynamically adjust authentication requirements based on risk, only requiring additional verification when anomalous circumstances are detected.
4. Privileged Access Intelligence
Privileged accounts represent particularly high-risk targets. AI-driven systems provide enhanced monitoring of privileged sessions by:
- Recording and analyzing privileged user activities
- Detecting unusual command sequences
- Identifying potential privilege abuse
- Monitoring sensitive resource access
- Alerting on policy violations in real-time
This capability is crucial for maintaining security in environments with extensive privileged access requirements such as DevOps and cloud infrastructure management.
Real-World Applications and Benefits
Threat Detection and Response
AI-driven anomaly detection dramatically improves threat detection capabilities. A 2023 study by Ponemon Institute found that organizations with advanced AI security tools detect and contain breaches 74% faster than those without such capabilities. This rapid detection can mean the difference between a minor security incident and a catastrophic data breach.
These systems can identify sophisticated attacks including:
- Account compromise and credential theft
- Insider threats and data exfiltration attempts
- Advanced persistent threats (APTs)
- Supply chain compromises
- Zero-day exploits targeting identity systems
Compliance and Audit Efficiency
Organizations facing regulatory requirements benefit from AI-driven anomaly detection through:
- Continuous monitoring of access compliance
- Automated detection of segregation of duties violations
- Enhanced audit trails with anomaly annotations
- Risk-based certification and access review
- Proactive identification of compliance gaps
For organizations in highly regulated industries like healthcare or financial services, these capabilities are invaluable for maintaining compliance with regulations like HIPAA, SOX, or FISMA.
Operational Efficiency
Beyond security benefits, AI-driven anomaly detection improves operational efficiency by:
- Reducing manual review requirements for access certifications
- Decreasing false positive alerts that drain security resources
- Enabling risk-based access governance
- Streamlining investigation workflows
- Supporting zero-trust architecture implementation
According to a recent study by Enterprise Management Associates, organizations implementing AI-enhanced IAM solutions reported a 35% reduction in security analyst workloads related to identity management and a 47% decrease in time spent on access certification processes.
Implementing AI-Driven Anomaly Detection: Key Considerations
Data Quality and Collection
The effectiveness of AI anomaly detection depends heavily on data quality. Organizations must ensure:
- Comprehensive logging of identity-related events
- Consistent data formats and normalization
- Sufficient historical data for baseline establishment
- Integration across diverse identity repositories
- Proper data retention policies
Integration with Existing IAM Infrastructure
AI-driven anomaly detection should complement and enhance existing identity management solutions, not replace them. Key integration points include:
- Identity governance and administration (IGA) systems
- Privileged access management (PAM) platforms
- Single sign-on (SSO) solutions
- User provisioning workflows
- Access certification processes
Avatier’s Identity Anywhere platform provides comprehensive integration capabilities that allow organizations to enhance their existing identity infrastructure with advanced AI capabilities while maintaining a unified management approach.
Privacy and Ethical Considerations
Organizations implementing AI-driven anomaly detection must address privacy concerns by:
- Ensuring compliance with relevant data protection regulations
- Implementing appropriate data minimization strategies
- Maintaining transparency about monitoring practices
- Establishing clear policies for investigation procedures
- Incorporating privacy-by-design principles
Continuous Learning and Tuning
AI systems require ongoing attention to maintain effectiveness:
- Regular model retraining to adapt to organizational changes
- Feedback loops to incorporate investigation outcomes
- Performance monitoring of detection accuracy
- Adjustment of sensitivity thresholds based on risk tolerance
- Periodic review of detection rules and algorithms
Future Trends in AI-Driven Anomaly Detection for IAM
Predictive Identity Security
The future of anomaly detection is moving from reactive to predictive approaches. Advanced AI systems are beginning to predict potential security incidents before they occur by identifying patterns that typically precede attacks. This shift from detection to prediction represents the next frontier in identity security.
Autonomous Response Capabilities
As AI systems mature, we’re seeing increased adoption of autonomous response capabilities that can:
- Automatically adjust authentication requirements based on risk
- Temporarily restrict access when suspicious behavior is detected
- Isolate potentially compromised accounts
- Initiate additional verification challenges
- Deploy honeypot resources to track attacker behavior
Extended Identity Intelligence
The scope of AI-driven anomaly detection is expanding beyond organizational boundaries to include:
- Supply chain identity risk monitoring
- Cross-organizational threat intelligence sharing
- Integration with external threat feeds
- Monitoring of identity risks across federated environments
- Detection of compromised credentials on the dark web
Conclusion: The Imperative for AI-Driven Anomaly Detection
As identity becomes the primary security perimeter in modern enterprises, traditional IAM approaches are insufficient to address evolving threats. AI-driven anomaly detection represents not just an enhancement but a fundamental shift in how organizations approach identity security.
The ability to continuously monitor, learn, and adapt to changing behaviors enables security teams to detect sophisticated attacks that would otherwise remain invisible until significant damage occurs. Organizations that embrace these technologies gain a significant advantage in threat detection, compliance management, and operational efficiency.
As attack vectors grow more sophisticated and regulatory requirements more stringent, AI-driven anomaly detection will become an essential component of enterprise security architecture. Organizations should begin planning their implementation strategy now to ensure they stay ahead of emerging threats and maintain robust protection of their critical assets.
By integrating AI-driven anomaly detection with comprehensive identity and access management solutions, organizations can create a robust defense system that continually evolves to address new threats while maintaining usability for legitimate users – the ultimate goal of any effective security program.